Parkinson’s disease (PD) is a neurological disorder characterized by the degeneration of dopamine-releasing regions in the brain. The loss of dopamine leads to a chronic and progressive decrease in movement, coordination, and cognition. While there is no cure, an early diagnosis promotes treatment efficacy and prolongs a higher quality of life.
Problems with stereological methods
Traditional stereological methods in PD research are time-consuming and involve tedious manual work, sometimes leading to error-prone analysis. Extrapolating data from samples also involves inter- and intra-observer bias, meaning final conclusions from varying samples can vary greatly between researchers and projects.
Artificial Intelligence (AI) -assisted image analysis increases the speed and scalability of projects, saving researchers valuable time to focus their efforts on more complex and higher-level challenges. Large data management and analysis consistency eliminate variation from random sampling, leading to improved statistical accuracy and reproducible results for future PD research.
Read more about how deep learning AI is a promising alternative to stereology →
Joan Compte, a predoctoral researcher at Vall d'Hebron Instituto de Investigación, has implemented AI models to save time in his work using Aiforia® Create. We interviewed him on the use of AI in PD research and his experience using Aiforia’s software without prior coding knowledge.
Why did you decide to use AI for your current research project?
"We decided to use AI for our projects because cell counting (of whatever staining) is very time-consuming. Besides, stereological methods provide just estimated numbers, which can lead to slightly error-prone analysis. Additionally, some stainings are very faint and difficult in the manual procedure. AI, like the one of Aiforia, helps to get rid of all these drawbacks and ensures a robust and efficient method to standardize and accelerate object counting."
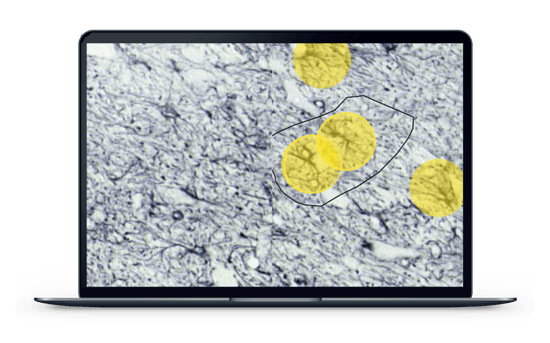
How did you find getting started with Aiforia Create?
"Working with Aiforia is very accessible for every user. Without any previous knowledge of AI or programming/code, the user can directly start training a specific algorithm for any of his/her objects. The interface is very intuitive and it gets very simple to begin accumulating hundreds of annotations. Besides, the annotation assistant tool makes the first proto-algorithms gain a lot of quality very quickly. On top of it, customer care of Aiforia is always ready to help with whatever inquiry and always give the best solutions."
"Aiforia makes life much simpler! Countings that could last for months are performed in seconds once your algorithm is ready. Besides, the different available tools optimize the whole procedure, reaching high-quality algorithms in just 1-2 months."
Read more neuroscience case studies:
References
Mosarrat, R. et al. (2019, February 14). Early detection of Parkinson’s disease using image processing and artificial neural network. IEEE Xplore. https://doi.org/10.1109/ICIEV.2018.8641081
Schmitz, C. & Hof, P. (2005). Design-based stereology in neuroscience. Neuroscience, 130(4), 813-831. https://doi.org/10.1016/j.neuroscience.2004.08.050
Souza, J. et al. (2018, April 24). A new approach to diagnose Parkinson’s disease using a structural cooccurrence matrix for a similarity analysis. Computational Intelligence and Neuroscience. https://doi.org/10.1155/2018/7613282