Alzheimer’s disease (AD) is the most common cause of dementia, attributed to its characteristic symptoms of brain shrinkage and cell death. Onset of the diseases is thought to be due to abnormal buildup of amyloid-β (Aβ), a protein in the brain, creating plaques and leading to neurodegeneration. Similarly, Aβ buildup can cause cerebral amyloid angiopathy (CAA), a condition which significantly increases dementia and stroke risk.
Training AI to identify important biomarkers
Traditional methods for neuropathological assessments of diseases often rely on visual assessments of stained tissue sections. The semiquantitative nature of the scoring process can lead to large variations between pathologists and institutions. Artificial intelligence has immense potential to improve medical image analysis and data management.
By utilizing whole slide imaging (WSI) of histological sections, AI models can effectively quantify histological objects like Aβ plaques, enhancing research efforts for both AD and CAA. Professor Susanne van Veluw and Dr. Valentina Perosa at the Massachusetts General Hospital, Department of Neurology have done exactly this with Aiforia® Create.
The researchers trained AI models to identify some of the most characteristic markers of AD and CAA, including Aβ plaques, iron and calcium deposition, reactive astrocytes, microglia, and fibrin extravasation, without the need to code or use dedicated hardware. We interviewed Susanne and Valentina to learn more about their publication and experience using Aiforia.
Describe the Alzheimer’s disease research project you used AI for
The van Veluw lab used Aiforia to develop deep learning-based AI models that identify some of the most characteristic histopathological markers of Alzheimer’s disease and cerebral amyloid angiopathy. So far, the deep learning-based models have been trained to differentially detect parenchymal amyloid β (Aβ)-plaques, vascular Aβ-deposition (CAA), iron and calcium deposition, reactive astrocytes, microglia, and fibrin extravasation. We have been using these measures in several projects, for example, to explore the association between neuroinflammation and Aβ pathologies and to confirm the relationship between iron-positive cells and CAA. Further work is ongoing in order to quantify more markers and investigate their crosstalk.
How is the AI model performing compared to using traditional methods?
Previously, we have mostly relied on semi-quantitative scores to describe histopathological markers of AD and CAA. Our recent work showed that the deep learning-based measures are consistent with experts’ observations for CAA, Aβ-plaques, and iron-positive cells. Moreover, we validated the Aiforia® Platform, which showed good to excellent agreement between human observers and the AI.
What do you think makes Aiforia unique in Alzheimer’s disease research?
The workflow enabled by Aiforia is easy for researchers with pathological expertise to implement, without the need to directly interact with a code, and is customizable to the research questions. This characteristic makes AI in histopathology more accessible. More generally, AI provides objective, continuous measures that allow the use of a greater variety of statistical models, such as linear mixed effects models. It can potentially also facilitate harmonization across datasets and centers, which opens new avenues for the relevance of histopathology in Alzheimer’s disease and CAA research.
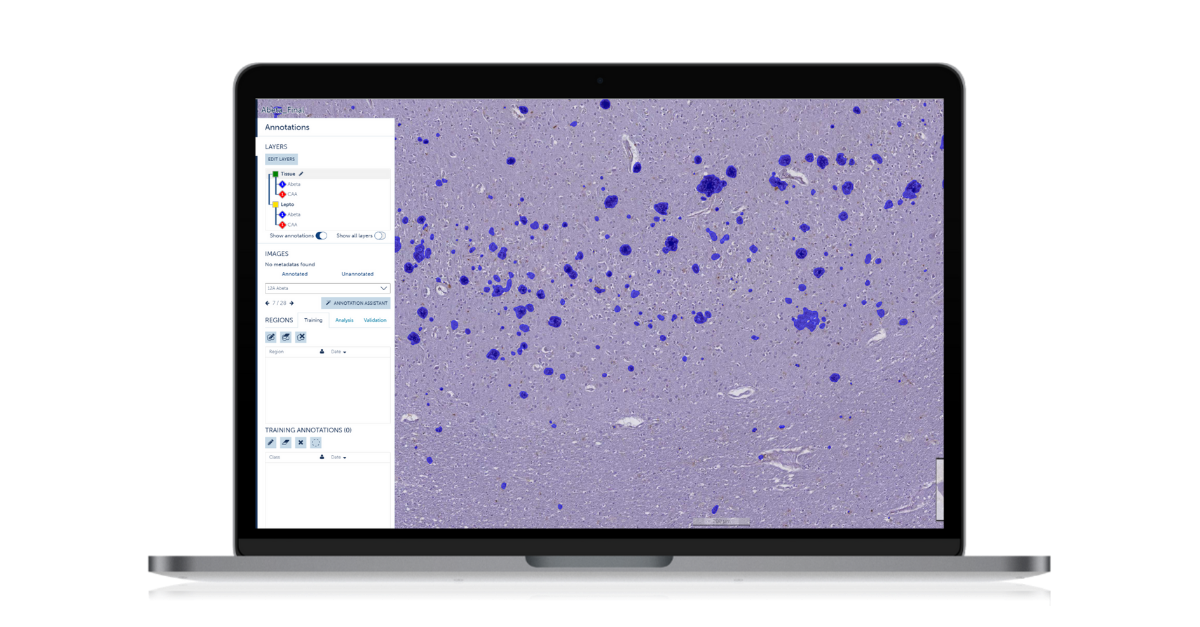
How was your experience using the Aiforia software?
The Aiforia® Platform is easy to use, and training a new AI model is relatively straightforward. Clarification and help are readily provided by the Aiforia team when needed so that the work can progress even if small obstacles should be encountered. The platform appears to be in constant development, allowing it in the future to save even more time in the training and analysis process.
Learn more about Aiforia's research solutions →
References
DeTure, M. & Dickson, D. (2019, August 2). The neuropathological diagnosis of Alzheimer’s disease. Molecular Neurodegeneration, 14(32). https://doi.org/10.1186/s13024-019-0333-5
Dietmar, R. et al. (2008, March 28). Cerebral amyloid angiopathy and its relationship to Alzheimer’s disease. Acta Neuropathol 115, 599–609. https://doi.org/10.1007/s00401-008-0366-2